A generalized framework for modeling mass transfer in two-phase, stirred tank bioreactors to aid the scale-up process and get important drugs to market faster.
In 2020, the pressure was on for major pharmaceutical companies like Pfizer. To respond to emerging viruses and variants, the demand for the rapid scale-up of biologic antibody production processes was high—and the need for high fidelity tools to assist in the development and scale-up of drug products had never been greater.
To take on this challenge, Pfizer turned to next-gen, GPU-native CFD software.
With M-Star CFD, Pfizer built digital twins to perform laboratory experiments on a computer, an approach that proved to accelerate the speed of manufacturing innovation and minimize the scope of the required supporting experimental studies.
The following case study follows findings from research featured in Chemical Engineering Science, 2021.
Authors: Hooman Farsani, Johannes Wutz, Brian Devincentis, John A Thomas, Seyed Pouria Motevalian
About Pfizer
Pfizer is an American multinational pharmaceutical and biotechnology corporation headquartered in New York, NY that innovates across a number of therapeutic areas, including internal medicine, rare disease, inflammation and immunology, oncology, and vaccines.
“Predictive tools for understanding the behavior of the microbioreactor a priori can reduce the number of required experiments while potentially streamlining the scale-up process.”
Problem
Microbioreactors play a pivotal role in making biologic medicines. This is because performing development and quality characterization studies at small-scale is more cost-effective than testing at pilot scales.
However, scientists still have to conduct a large set of experiments due to the extensive range of operating parameters. And once a manufacturer identifies an appropriate set of operating conditions, they must reproduce the fluid mechanical environment at larger scales—a complex feat due to the fact that not all system parameters can be harmonized across all system scales.
Did You Know?
10 of the top 15 selling drugs worldwide were made in a bioreactor.
Under normal circumstances, this is already a significant pain point for drug developers. But it’s even more urgent today with the call for faster vaccine development and production.
So, Pfizer researchers needed a way to understand and predict the behavior of the microbioreactor in hopes of reducing the number of required experiments and streamlining the scale-up process.
Solution
To predict fluid flow and transport inside two-phase bioreactors—and successfully scale-up production and bring important compounds to market faster—Pfizer turned to next-gen, GPU-enabled CFD software that solves lattice-Boltzmann-based transport algorithms with GPU resources.
System Configuration
- Vessel Configuration
- Agitation Speed
- Fluid Properties
- Sparge Flow Rate/ Composition
- Initial Bubble Size
- Headspace Properties
Simulation Parameters
- Grid Spacing: Must Satisfy Grid Convergence
- Timestep: Must Ensure Compressibility
- Smagorinsky Coefficient: Not Adjusted
Transport Relationships
- Free Surface Transport
- Bubble Surface Transport
- Bubble Break-Up Condition
- Daughter Volume Fraction
- Coalesce Criteria
Computational Context
Most two-phase bioreactor simulations developed over the last two decades have combined a timeaveraged representation of the flow field with a time-averaged representation of the bubble size distribution. These simplifications reduce the computational complexity of the problem, making twofluid bioreactor simulations possible on CPU hardware.
However, the reduction in computational complexity introduces challenges related to:
- Parameter tuning
- Correlation specification
- Model trustworthiness
Over the last few years, the maturation of GPU-based computing hardware and massively parallelizable physics algorithms have presented a step-change in generally available simulation capabilities.
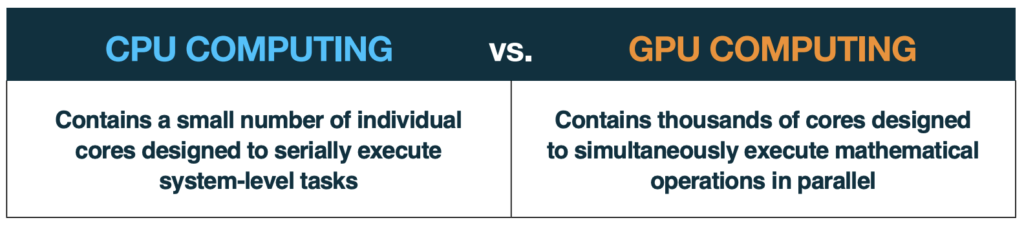
Over the last few years, the maturation of GPU-based computing hardware and massively parallelizable physics algorithms have presented a step-changeThe hardware cost of both systems is comparable, but a massively parallel algorithm running on a GPU workstation will advance orders-of-magnitude faster than what could be realized in a similarly priced CPU environment. Because of this improvement in performance, we can roll back many of the simplifying physics assumptions originally introduced to make CPU-based models practical.in generally available simulation capabilities.
“The net effect of these advances is a theoretically self-consistent formulation for building models, which requires little to no reparameterization across scale or operating condition.”
By taking advantage of these advanced tools, Pfizer was able to develop a physics-based, in-silico modeling approach with digital twins that provided a roadmap for process scale-up.
Results
The approach was validated by studying an Ambr® 15 microbioreactor over a range of operating conditions. Using this model, Pfizer researchers were able to decompose gas transfer to the fluid into contributions from the free surface and individually sparged bubbles, as well as identify the sensitivity of the mass transfer coefficient around each bubble to the local fluid mechanics. Insights garnered by this high fidelity approach not only provided a roadmap for process scale-up but also reduced the number of experiments needed to predict fluid flow in microscale bioreactors.
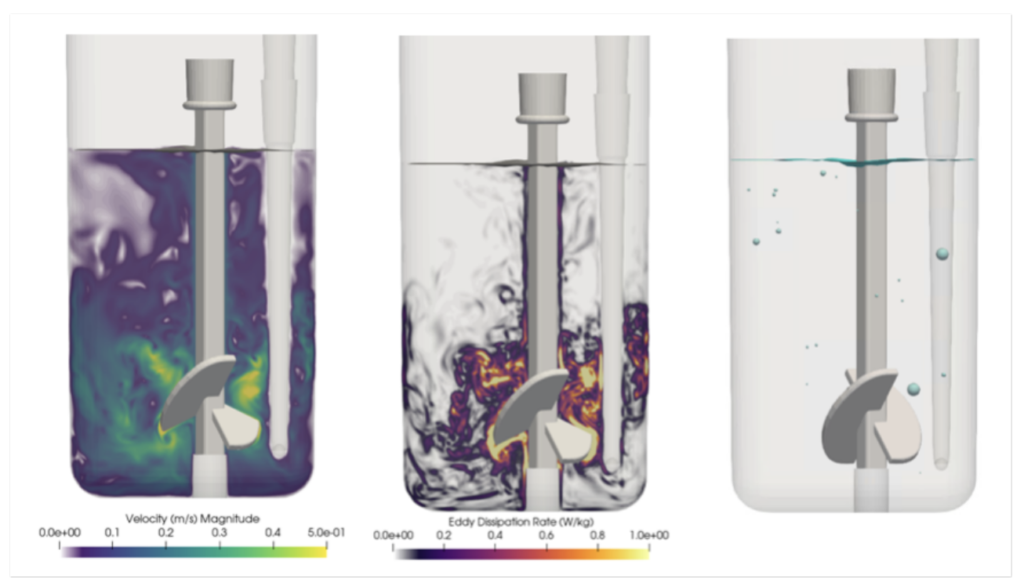
These in-silico engineering tools are one of the key reasons why Pfizer is able to bring drug products to market even faster.